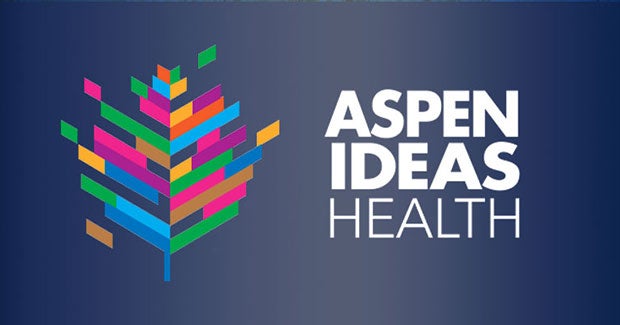
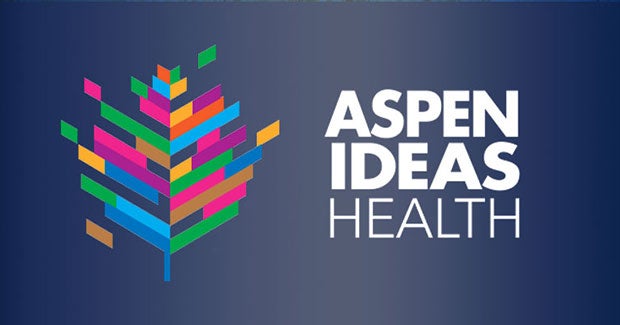
Take Steps Now or it Could be a Long Wait to Fulfill AI’s Promise
More accurate diagnoses in less time. More effective treatments as the first option. Clinicians with the ability to practice at the top of their license and the time to connect meaningfully with patients. These are just a few of the benefits artificial intelligence could bring to health care. Yet, they’re not as close as you may think.
What’s holding us back — and how to overcome these barriers — was one of many topics explored at the recent Aspen Ideas Health, “where health care's biggest challenges meet its biggest thinkers. "AHA members and leaders participated as speakers in a number of sessions over the course of the three-day festival, drawing more than 1,200 attendees from 22 countries.
Here’s what we learned about AI in health care:
-
AI solutions solve mostly narrow problems. AI in health care comprises many specific solutions for a variety of specific-use cases. For example, AI might be used to predict and identify patients most at risk for sepsis, or a COPD patient likely to readmit. While there’s value for each use case, it’s not exactly optimized for providers who are trying to integrate dozens, and perhaps hundreds, of specific solutions into care delivery.
What to do about it: Before bringing in your next AI solution, hospital leaders should prioritize the use cases with the highest impact, not just the loudest internal champion. Leaders also need to make sure the solution will integrate into the electronic health record system and existing workflows.
-
Health data are too fragmented. Health data live in many different IT systems, and not all systems talk to one another within a provider organization, let alone across organizations. In one session, experts agreed that AI is advanced enough today to guide differential diagnoses, which would particularly help patients with rare diseases that aren’t easily diagnosed. But, “data [are] not systematically accessible in a way where you can layer in [machine learning] effectively,” said Lizzie Dorfman of Google AI.
What to do about it: Experts say we can get to AI-informed diagnoses in the next decade (longer than we would have guessed), but a lot of data consolidation within organizations and data sharing across organizations must happen first. While giving patients control of their own data may move this process along, all organizations that touch the delivery system — from hospitals, to insurers to pharmaceutical companies — need to explore data sharing and partnerships in a way they haven’t before.
-
Fee-for-service payments. Certain existing AI use cases could reduce unnecessary care. For example, using image-recognition AI to identify what is likely cancerous could reduce the number of patients who need biopsies. But finding an organization to pilot this type of a solution was a challenge for one AI company. To find an organization that was excited about doing fewer biopsies, they needed an organization that was already at risk for a majority of its patients, and had mostly employed physicians.
What to do about it: Although the pace of the transition to value-based payment and taking on risk varies in hospitals across the country, all hospitals should continue to position themselves for success under value-based payment by implementing new care models that improve outcomes while reducing cost. A recent AHA Center for Health Innovation report, “ Evolving Care Models: Aligning Care Delivery to Emerging Payment Models,” provides an overview of the successes and challenges providers have experienced in aligning care delivery models with emerging payment models.