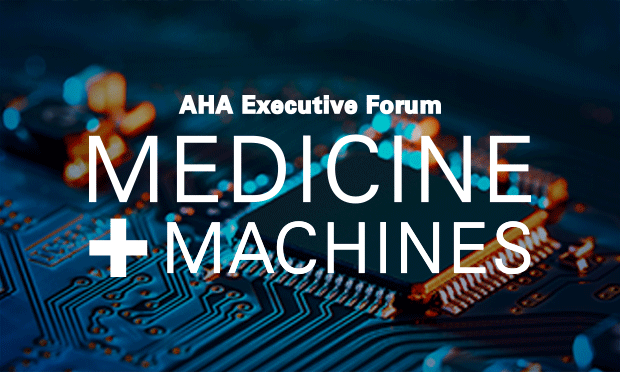
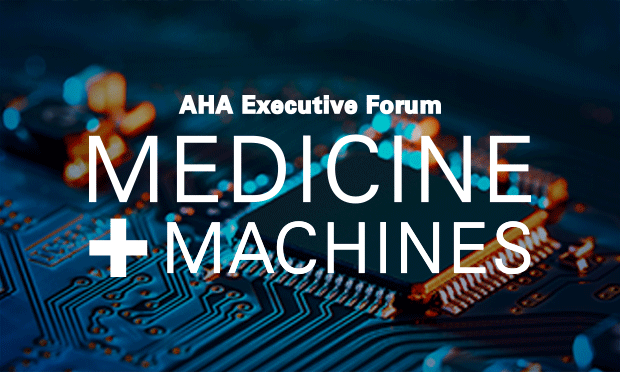
5 Ways AI is Improving Care Delivery
Hospitals and health systems are deploying artificial intelligence to transform care delivery. So, what do some of these applications look like? At the recent AHA Executive Forum in Chicago, five health systems shared ways they are addressing pressing challenges through the use of algorithms, predictive analytics and machine learning.
Personalized care management for total hip and knee replacement
Hospital for Special Surgery, New York City, has designed a personalized health management tool that takes a patient from initial contact to a smart patient intake process — an interactive, personalized health assessment including history, risk factors, treatment goals, preferences and premature rupture of membranes.
Catherine MacLean, M.D., Ph.D., chief value medical officer at the hospital’s Center for the Advancement of Value in Musculoskeletal Care, said the patient data are run through an AI model to predict diagnosis, complications and outcomes for various disease treatment options. These options are presented along with patient-specific predictions on the likelihood of improvement, achievement of goals and complications. The patient then is provided with a portal for secure ongoing communication with the provider.
Predicting respiratory failure risk
Parsa Mirhaji, M.D., Ph.D., director, Center for Health Data Innovations at Montefiore Health System, and research associate professor of systems and computational biology at the Albert Einstein College of Medicine, discussed the development of a classification algorithm consisting of many decision trees that can detect early signs of acute respiratory failure up to 48 hours in advance. The model, Accurate Prediction of Prolonged Ventilation, or APPROVE, was trained on 58,000-plus admissions to Montefiore using more than 50 variables and more than 200 independent decision trees. It has been validated on 28,088-plus encounters and is now integrated into the electronic health record.
When APPROVE detects that a patient might be at risk or reaches a predefined threshold, it issues an alert to clinicians in the form of a best-practice advisory, a pop-up message on a patient’s EHR with a checklist of steps intended to prevent or mitigate acute respiratory failure. This is an example of operationalizing predictive analytics at the bedside.
AI and kidney disease: changing the paradigm
Girish Nadkarni, M.D., assistant professor of medicine, division of nephrology and clinical director of the Charles Bronfman Institute for Personalized Medicine at Mount Sinai Health System, explained how his organization is using AI-enabled clinical decision support tools for improved kidney disease risk assessment and clinical care.
Using blood markers, genetic factors and patient information from the EHR, the AI machine learning engine co-developed with RenalytixAI, an AI systems company, creates a score and risk category for patients and providers along with a clinical interpretation and care pathways. This application supports physician decision-making by improving identification, prediction, risk stratification and the clinical care of patients with progressive kidney disease from mild loss of kidney function to kidney failure and dialysis.
Detecting and preventing sepsis
HCA Healthcare has developed an AI-based decision support tool, Sepsis Prediction and Optimization of Therapy (SPOT), for the early detection of sepsis. Jonathan B. Perlin, M.D., Ph.D., MSHA, MACP, FACMI, president of clinical services and chief medical officer, describes SPOT as a smoke detector of sorts for sepsis. The algorithm is embedded in the EHR and combines patient demographic data and medical history with continuous monitoring for signs of sepsis and key elements of clinical care. When the signs of sepsis are detected, an alert is sent to caregivers for rapid response.
For every hour of delayed diagnosis and therapy, sepsis mortality increases up to 8%. The AI tool, together with the use of evidence-based clinical interventions, helped save an estimated 8,000 lives in the last five years. SPOT was implemented in 53 hospitals over 21 months, compared with the average of 17 years for research evidence to reach clinical practice.
AI chatbot for outpatient chemotherapy
Roy Rosin, chief innovation officer at Penn Medicine, said that on average, medication adherence among outpatient chemotherapy patients was only 50% with a high number of unnecessary emergency department visits. Penn Medicine saw an opportunity for improvement and developed Penny, an AI chatbot, to assist patients with their outpatient chemotherapy regimens. Penny provides step-by-step personalized guidance.
The adaptive rules engine enables Penny to respond to patient-reported side effects for rapid symptom management. Briefings are sent to providers before in-person office visits to optimize interactions. Currently, Penny is being piloted and projected to handle 78% of the calls an average patient makes and to prevent unnecessary ED visits.