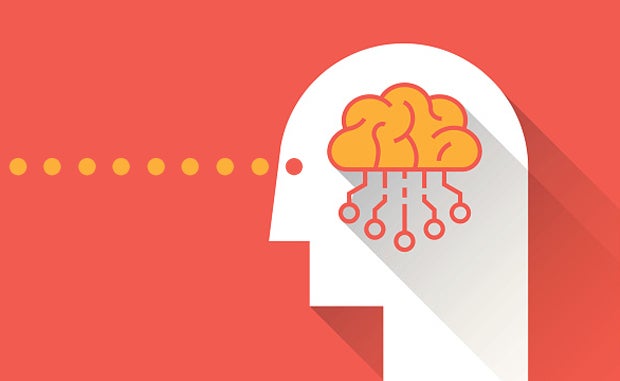
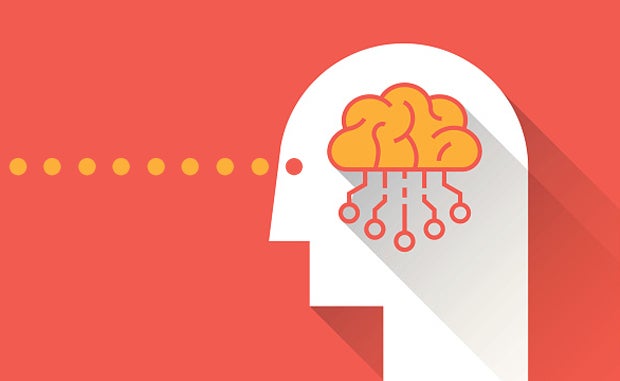
Study Finds New Commercial AI Devices Often Lack Key Performance Data
Enthusiasm for and adoption of commercial artificial intelligence (AI) tools to aid clinical decision-making and patient care have been accelerating at a rapid pace. A new study, though, cautions that critical information often is missing from the Food and Drug Administration (FDA) clearance process that could measure how these devices actually work in patient care.
The study, published in the research journal Nature Medicine, notes that while the academic community has started to develop reporting guidelines for AI clinical trials, there are no established best practices for evaluating commercially available algorithms to ensure their reliability and safety.
And while the FDA is working on regulations for how commercial AI devices should be evaluated, provider organizations will have to analyze these tools based on limited data. What concerns authors of the Nature Medicine article is whether the information submitted by manufacturers is sufficient to evaluate device reliability.
Most of the cleared AI tools are designed to work as triage devices or to support clinician decision-making. In their review of 130 FDA-cleared AI devices, the researchers found that 126 were based on retrospective data, i.e., data collected from clinical sites before evaluation. And none of the 54 high-risk devices were evaluated by prospective studies. This is significant because prospective studies are needed to show how devices actually work with physicians’ and hospitals’ data systems.
“A prospective randomized study may reveal that clinicians are misusing this tool for primary diagnosis and that outcomes are different from what would be expected if the tool were used for decision support,” the study notes.
Lack of Data on Effectiveness by Demographics, Sites
Most disclosures did not include the number of sites used to evaluate the AI devices nor whether the tools had been tested to see how they perform in patients of different races, genders or locations. This shortcoming in the current review system can make a significant difference in how AI tools perform, the study notes. Also, it wasn’t clear how many patients were involved in the testing of an algorithm. Of the 71 devices in which this information was shared, companies had evaluated the AI tools in a median of 300 patients. Having more thorough public disclosures would be beneficial to provider organizations who need to identify potential vulnerabilities in how a model performs across different patient populations.
How the FDA Is Responding
In January, the FDA released its first AI and machine learning (ML) action plan, a multistep approach to further the agency’s management of advanced medical software. The plan outlines the FDA’s next steps toward advance oversight for AI-ML-based software as a medical device and will further develop the proposed regulatory framework.
The agency acknowledges that AI-ML-based devices have unique considerations that require a proactive, patient-centered approach to their development and utilization that takes into account such issues as usability, equity, trust and accountability. The FDA is addressing these issues by promoting the transparency of the devices to users, and to patients more broadly. Comments from the health care field identified the need for manufacturers to clearly describe the data that were used to train the algorithm, the relevance of its inputs, the logic it employs (when possible), the role intended to be served by its output, and the evidence of the device’s performance.
3 Tools to Navigate AI Decision-Making
These AHA Market Insights reports can help leaders navigate the AI landscape:
- “Selecting the Right AI Vendor Partner,” an AHA member-only resource, provides key questions to ask vendors, whether organizations are working on a homegrown AI project or outsourcing AI projects.
- “AI and Care Delivery” helps leaders successfully integrate AI-powered technologies to improve outcomes and lower costs at each stage of care.
- “17 Questions for Leadership Teams,” another AHA member-only tool, provides strategic questions leaders should consider when integrating AI technologies into care delivery.