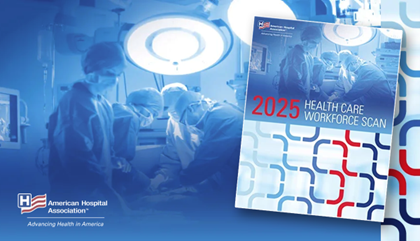
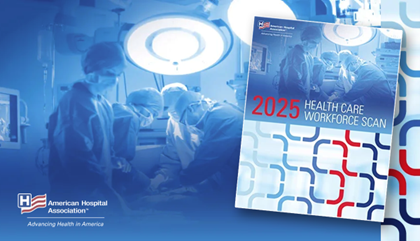
3 Ways to Reimagine Care Delivery to Support Your Workforce
Hospitals and health systems continue to focus on enhancing support and fostering resilience among a thinly stretched, post-pandemic workforce. Facilities across the country are investing in artificial intelligence (AI) technology and tech skills development to alleviate burden and enhance flexibility. Many also are reimagining care delivery models.
The recently released AHA 2025 Health Care Workforce Scan provides a comprehensive review of these and other issues, including:
- Engaging clinicians in technology strategies.
- Boosting access through partnerships, training and upskilling.
- Rethinking engagement to build a more flexible, engaged workforce.
The workforce scan is based on the latest reports, studies and other data sources and offers valuable insights into the workforce landscape, as well as practical guidance from experts and peers to help your organization navigate the future of the health care workforce.
Embrace Technologically Integrated Care Models
Amid high provider burnout and financial pressure, hospital leaders are embracing technology, including AI, as a transformative tool. More established technologies like virtual care, telesitting and remote patient monitoring continue to support front-line staff and expand access in integrated care models. These tools show promise in boosting staff retention, efficiency and care quality.
AI applications are expanding rapidly across many clinical and operations areas. Ohio-based University Hospitals Health System, for example, has deployed an AI platform with more than 30 care pathways to quickly identify conditions like pulmonary embolism, coronary artery calcification and intracranial hemorrhaging. Others are using AI to produce faster results in cancer screenings. And generative AI, while still being evaluated for its accuracy and optimal uses, is reducing clinician workloads in clinical documentation and creating patient summaries.
Leaders have found value through other applications, including:
- Personalizing treatment: Machine learning can automate complicated statistical work and discover which characteristics indicate that a patient will have a particular response to a given treatment.
- Identifying infection risks: AI applications can help predict when a patient’s immune system may have an overwhelming and potentially dangerous response to an infection. AI also can assess dozens of variables at one time, track a patient’s vital signs to detect sepsis risk earlier and enable clinicians to respond more quickly.
- Enhancing disease detection: AI can help automate time-consuming tasks like tracing tumors and measuring amounts of fat and muscle.
3 Technologically Integrated Care Models to Explore
Sentara Health | Norfolk, Virginia
Research shows that nurses who feel overworked or unable to finish their tasks are more likely to burn out and quit. Sentara Health developed the Patient Acuity Nursing Tool (PANT) to improve nurse-patient assignments by objectively assessing nursing workloads and patient acuity.
Traditional methods relied on the clinical judgment of charge nurses and patient census data, often leading to imbalanced workloads. The PANT algorithm, created by an interdisciplinary team at Sentara, integrates real-time patient data to distribute nursing tasks equitably. The health system has implemented it across 100 inpatient units, supported by training materials and orientation programs. The tool helps charge nurses make more informed assignments, reduces nurse burnout and enhances patient care by providing a validated measure of nursing workload.
Stanford Medicine | Palo Alto, California
Stanford Medicine researchers implemented an AI-based model at Stanford Hospital that predicts patient deterioration and facilitates better collaboration between physicians and nurses. The model analyzes real-time patient data every 15 minutes and sends alerts to care teams when a patient is at risk of decline.
While the model isn’t perfect, with about 20% accuracy in predicting actual deterioration events, it led to a 10.4% decrease in deterioration events among high-risk patients. The AI model serves as a communication facilitator, prompting important conversations among clinicians that might not otherwise occur due to busy schedules.
Ochsner Medical Center | Jefferson, Louisiana
The Medical Center used data science to improve patient safety. It created a neural network model using information from 250,000 hospital stays to predict Clostridioides difficile infections. The data revealed that stomach acid-reducing medicines increased infection risk more than expected.
Now, Ochsner’s system flags high-risk patients, giving pharmacists the opportunity to advise its doctors on medication use (often suggesting using these medicines less or stopping them). This new approach cut C. difficile infections by half, prevented 166 infections and saved $4 million in two years.
3 Things to Think About
- How will you integrate AI within your existing technology infrastructure, particularly the electronic health record?
- How will you monitor and evaluate the performance and impact of AI technologies over time, including potential unintended consequences or biases?
- How can you proactively identify and alleviate issues that add to clinical burden?