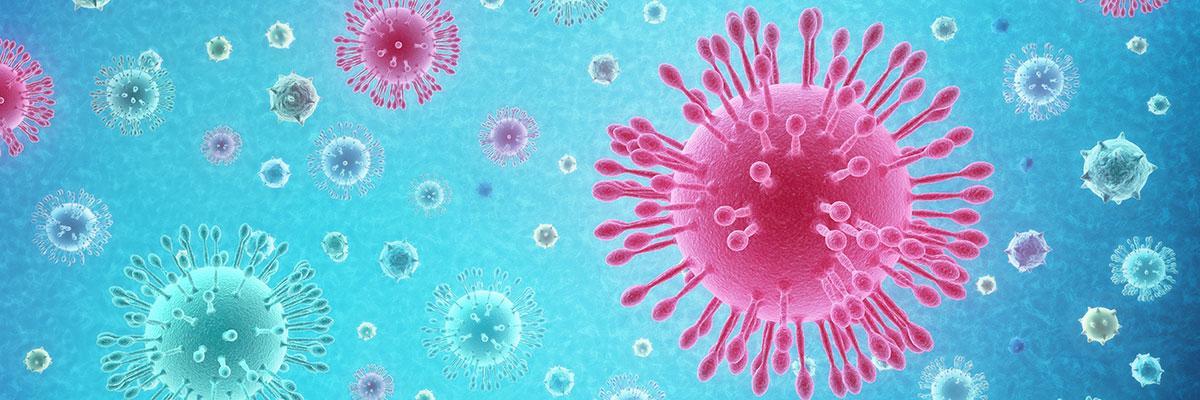
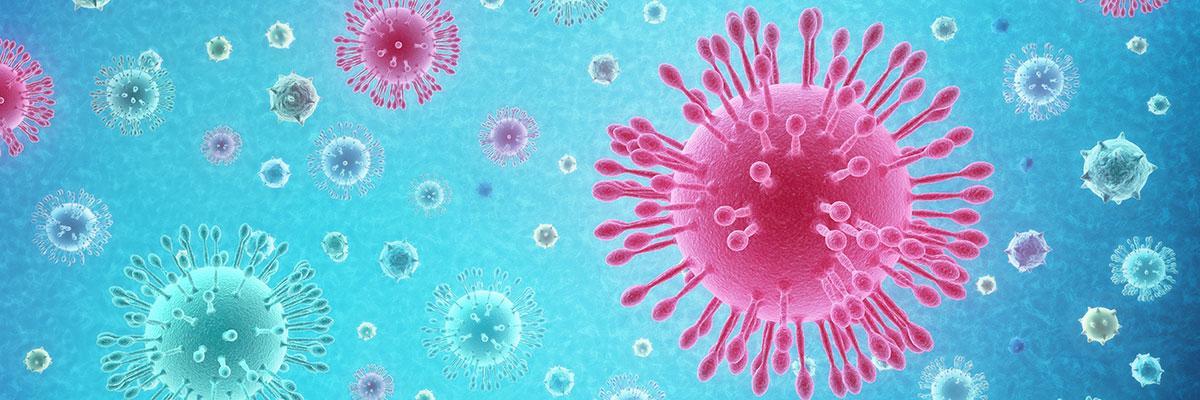
A Compendium of Models that Predict the Spread of COVID-19
COVID-19 Models: Forecasting the Pandemic’s Spread
April 2020
With our evolving understanding of the details of COVID-19 infection and transmission, the impact of socialdistancing measures and other factors, hospitals and health systems face complex forecasting challenges.
A growing number of models have been developed by health care systems, academic institutions, consulting firms and others to help forecast COVID-19 cases and deaths, medical supply needs, including ventilators, hospital beds and intensive care unit (ICU) beds, timing of patient surges and more.
We recognize that all forecasting is imperfect and that models often change as data are revised to reflect evolving conditions in the population. The American Hospital Association (AHA) offers this resource in response to members’ interest in tools and resources that can help them make decisions that are consistent with their organizations’ values and community needs.
The Case Projection and Capacity Planning models are all predicated on some assumptions about the current status of the disease, how quickly it spreads (e.g., doubles), and/or the degree of containment efforts being deployed. Those assumptions drive the rise, peak and decline in “the curve” — the number of COVID-19 cases, deaths or resources needed. The simpler models apply the user’s inputs on the local population and current status of COVID-19. The more sophisticated models allow the user to alter other parameters (e.g., changes in social distancing) that influence the curve. Some of the models match projected cases to existing capacity to estimate when and where a caseload surge will exceed capacity.
The Community Vulnerability Maps use social and economic population variables, such as density, travel distances to health care, underlying chronic illnesses, economic status, to highlight communities that are most at risk for contracting and/or succumbing to the disease.
The Case Mapping and Projection Tools provide a quick visual depiction of disease incidence at the national, state or county levels.
The following list of resources was updated on April 6 and is not exhaustive. It will continue to be updated periodically as additional models are assessed. These resources are classified into categories based on the extent of their modeling capabilities and how they can be used.
Please note that the tools listed do not necessarily reflect the views of the AHA, nor do they constitute advice from the AHA. Rather, they are meant to assist hospitals and health systems in making decisions for their own organizations.
Case Projection and Capacity Planning
NEW Harvard University — Regional Hospital Capacity Calculator
This model was developed by researchers at the Harvard Global Health Institute (HGHI) and the Harvard T.H. Chan School of Public Health, and was launched in collaboration with ProPublica, and in sync with news reports in The New York Times and “CBS Morning News.” The model provides projections for the U.S. and for each Hospital Referral Region (HRR).
Additional Details
- Data and assumption sources: The model combines data on hospital beds and population with estimates from recent research on estimated infection rates, proportion of people hospitalized (general med-surg and ICU), average lengths of stay (LOS), increased risk for people older than 65 and transmission rate. The data used to produce the estimates are available and can be downloaded from the HGHI site. It is not clear when the data were last updated.
- Estimation level: HRR.
- Degree of complexity: Easy. User inputs a city and receives results for the corresponding HRR using the default assumptions. [Note: User has to scroll down this page to see the bar charts.]
- Software platform/language: Web-based.
- Ability to change assumptions and run scenarios: No.
- Output: National heat maps showing HRRs and the percentage of occupancy levels under three different national infection rates (20%, 40% and 60%) and within three time periods (6, 12 and 18 months), as well as bar charts showing the same information for the chosen HRR.
- Methodology: Methodology and assumptions documentation.
NEW Qventus — Localized COVID-19 Model and Scenario Planner
The Qventus model provides localized projections based on the latest local estimates and research on COVID-19. The estimates are projected using a modified Susceptible-Exposed-Infectious-Recovered (SEIR) model that starts with local case counts and resource estimates. The model’s parameters are populated automatically with assumptions specific to the HRR of the selected hospital(s). Qventus continually updates the estimates and assumptions based on actual disease progression.
Additional Details
- Data and assumption sources: Default model assumptions such as current COVID-19 census, growth rate, percentage of infections reported, average recovery time and hospitalization rates come from multiple sources, including current county case counts, recently published research, Healthcare Cost and Utilization Project and Qventus clients.
- Estimation level: HRR.
- Degree of complexity: Easy. User inputs hospital name and receives HRR resultly using the default assumptions or the user’s own assumptions. Users also can download and share the output.
- Software platform/language: Web-based.
- Ability to change assumptions and run scenarios: Users can alter model scenarios (e.g., social distancing, improved LOS flow, bed capacity, staffing), market conditions (e.g., population and market share), hospital assumptions (e.g., ICU capacity and current COVID-19 census) and COVID-19 assumptions (e.g., doubling time and fatality rate).
- Output: Expected next seven-day admissions requiring medical-surgical only, ICU or ventilator and days until resource capacity is reached; expected total deaths over the next 45 days, projected cumulative med-surg and ICU occupancy, and ventilator and PPE utilization.
- Methodology: Methodology and assumptions documentation. Description of SEIR modeling: https:// www.idmod.org/docs/hiv/model-seir.html
NEW Rush University — Hospital Resource Calculator for COVID-19
The Rush University Center for Quality, Safety & Value Analytics forecasting tool provides state-level projections of confirmed COVID-19 cases and forecasts of hospital COVID-19 visits and resource needs including nonintensive care unit and ICU beds, ventilators and personal protective equipment (PPE). The tool allows users to choose from six growth models to predict the number of COVID-19 cases up to 60 days into the future, and also provides prior predictions from the 10 most recent days, allowing users to graphically assess how prior projections differ from the actual case counts in the past 10 days. It also provides the coefficient of determination as a measure of how close the predictions are to the data.
Additional Details
- Data and assumption sources: The model uses daily confirmed case counts from the Johns Hopkins University Center for System Science and Engineering’s GitHub repository, which in turn takes its data from multiple sources across the world such as the World Health Organization, Centers for Disease Control and Prevention and the Chinese Center for Disease Control and Prevention.
- Estimation level: State and hospital level.
- Degree of complexity: To forecast a hospital’s resource use (beds, ICU beds, ventilators and PPE), users would select a model type, choose the state in which the hospital is located and enter baseline information such as the hospital’s percentage of the state’s COVID-19 cases, the percentage of non-ICU and ICU cases, the percentage needing PPE, and critical and noncritical LOS.
- Software platform/language: Web-based.
- Ability to change assumptions and run scenarios: Yes. Users can select from six prediction models, specify forecast length and alter hospital admission and resource parameters.
- Output: Projected number of COVID-19 cases, daily bed needs by bed type (non-ICU and ICU), number
- Methodology: This tool offers six growth models: Susceptible-Exposed-Infected-Recovered-Dead (SEIRD), logistic, exponential, quadratic, cubic and Gaussian models. Instructions and descriptions of some of the models are provided and the Python source code is publicly available.
Penn Medicine — CHIME: The COVID-19 Hospital Impact Model for Epidemics
This model allows hospitals to enter information about their population and modify assumptions about the spread and behavior of COVID-19. It then runs a standard Susceptible-Infected-Recovered (SIR) model to project the number of new COVID-19 hospital admissions each day, along with the hospital census of COVID-19 patients. These projections then can be used to create best- and worst-case scenarios to assist with capacity planning. Penn Medicine is open-sourcing CHIME and making it available to the health care community.
Additional Details
- Data and assumption sources: The discrete-time SIR model has user inputs for population, the hospital’s market share, current COVID-19 hospitalizations, doubling time, severity and social distancing. It employs a combination of estimates from other locations (e.g., Wuhan, China; South Korea; Italy), informed estimates based on logical reasoning.
- Estimation level: Individual hospital or health care system.
- Degree of complexity: User interface with tutorial and documentation.
- Software platform/language: Web-based.
- Ability to change assumptions and run scenarios: Yes.
- Output: Projected new COVID-19 admissions per day (e.g., hospitalized, ICU, ventilator) and projected total COVID-19 patients per day for the same three cohorts. Projections for SIR patients.
- Methodology: SIR model documentation.
Health Catalyst — Capacity Planning Tool
This tool builds on Penn Medicine’s model. Health Catalyst added new features such as the ability to run multiple scenarios, store the scenarios on users’ local desktops, and then upload them again for later use. Additional features include predicted impacts of scenarios on hospital capacity (e.g., beds and ventilators).
Additional Details
- Data and assumption sources: Penn Medicine’s discrete-time SIR model. User inputs for population, market share, current COVID-19 hospitalizations, hospital capacity, doubling time, severity and social distancing.
- Estimation level: Individual hospital or health care system.
- Degree of complexity: User interface with tutorial and documentation.
- Software platform/language: Web-based.
- Ability to change assumptions and run scenarios: Yes.
- Output: Projected new COVID-19 admissions per day (e.g., hospitalized, ICU, ventilated) and projected total COVID-19 patients per day for the same three cohorts. Projections for SIR and bed capacity (e.g., total beds, ICU beds, ventilators).
- Methodology: Statistical model documentation and application guidance.
Cleveland Clinic — Hospital Impact Modeling
Also building on the Penn Medicine model, Cleveland Clinic’s model is available in GitHub, with documentation. It uses Penn’s SIR assumptions, but allows more flexibility to hold time periods constant and model various scenarios as well as update the curves, based on inputs such as changes in response measures. The model is Statistical Analysis System (SAS)-based and, in its current iteration, does not have a user interface. Users will need advanced SAS skills to alter assumptions.
Additional Details
- Data and assumption sources: This model extrapolates to several model types like the Susceptible-Exposed-Infected-Removed (SEIR) model. User inputs for population, market share, admission rate for COVID-19 on Day 0, COVID-19 infections on Day 0, social-distancing baseline and change, incubation period, recovered patients, recovery days, doubling time, ICU percentage, ventilator percentage, fatality rate and length of stay. Assumptions are based on the population studied in the Cleveland Clinic and SAS collaboration.
- Estimation level: Individual hospital or health care system.
- Degree of complexity: Complex SAS code with documentation. Geared to users with SAS experience.
- Software platform/language: SAS.
- Ability to change assumptions and run scenarios: Yes.
- Output: Daily projections of disease progression and hospitalization.
- Methodology: Statistical models documentation.
UW-IHME — COVID-19 Forecasting Tool
The University of Washington’s Institute for Health Metrics and Evaluation forecasting tool provides state-bystate information on anticipated number of beds needed, ICU beds needed and deaths related to COVID-19. Projections assume full social distancing through May. The tool does not permit hospital-level inputs and alternate scenarios.
Additional Details
- Data and assumption sources: Data on confirmed COVID-19 deaths by day from World Health Organization websites and local and national governments; data on hospital capacity and utilization for U.S. states from the AHA; and observed COVID-19 utilization data from select locations (e.g., Italy, China, Korea, and the U.S). Model forecasts peak death and resource usage dates, and daily deaths and hospital utilization (e.g., beds, ICU beds, ventilators) against capacity by state and for the U.S. over the next four months (until July), assuming full social distancing through May.
- Estimation level: Individual states and U.S.
- Degree of complexity: No user inputs, easy to comprehend.
- Software platform/language: Web-based.
- Ability to change assumptions and run scenarios: No.
- Output: Graphs showing peak death and resource utilization dates, daily total bed and ventilator needs and shortages, and projected deaths.
- Methodology: Statistical models (e.g., nonlinear mixed-effects model) documentation.
Stanford Medicine — Hospital Bed Projections
This COVID-19 ICU and floor-projection planning tool uses a facility’s own patient census numbers and hospital capacity. The model is designed to facilitate hospital planning with estimates of the daily number of intensive care beds, acute care beds and ventilators necessary to accommodate patients who require hospitalization for COVID-19 and how these compare to the available resources.
Additional Details
- Data and assumption sources: COVID-19 admissions are projected with the exponential growth model based on the inputs of the doubling time and the initial number of patients. The user inputs the census data of the ICU, floor and general medicine, along with daily admissions and hospital capacity parameters.
- Estimation level: Individual hospital or health care system.
- Degree of complexity: User interface has the option to upload facility’s daily census data or use built-in parameters.
- Software platform/language: Web-based.
- Ability to change assumptions and run scenarios: Yes.
- Output: Projected number of patients in ICU, non-ICU and on ventilators and days until capacity is exceeded.
- Methodology: Model explanation in more detail.
RAND Corporation — Critical Care Surge Response Strategies
In this report, the authors present a list of strategies for creating critical care surge capacity and estimating the number of patients accommodated, given the number of available critical care doctors and nurses, respiratory therapists, ventilators and hospital beds. They also document the development of a user-friendly, Microsoft Excel-based tool that allows decision-makers at all levels — hospitals, health care systems, states, regions — to estimate current critical care capacity and rapidly explore strategies for increasing it.
Additional Details
- Data and assumption sources: Formulas driven by user inputs — staff ratios, staff levels, additional personnel resources and patient capacity; model uses AHA data, American Medical Association data, and other data on critical care space, staff and supplies (e.g., ventilators, PPEs).
- Estimation level: Individual hospital, health care system, regional or state levels.
- Degree of complexity: Clear descriptions of required inputs.
- Software platform/language: Excel.
- Ability to change assumptions and run scenarios: Yes.
- Output: Total number of patients who can be cared for per shift by staff and resource type.
- Methodology: Limited documentation.
Community Vulnerability Maps
Jvion — COVID-19 Community Vulnerability Map
Clinical artificial intelligence company Jvion launched this public-facing tool, built on Microsoft Azure maps. It enables health care providers and communities battling the COVID-19 pandemic to identify the social determinants of health (SDOH) that put populations at greater risk, informing community planning and resource allocation to proactively mitigate the risk to vulnerable populations. The interactive map identifies populations down to the census block level that are at risk for severe outcomes upon contracting a virus like COVID-19.
Conduent — Locating At-Risk Populations Tool
Conduent Healthy Communities Institute specializes in providing SDOH data to organizations so that they can improve health in their respective populations. Their tool lets users track at-risk populations by state and county.
Case Mapping and Projection Tools
Oliver Wyman Consulting — COVID-19 Scenario Generator
This scenario generator from Oliver Wyman predicts the growth and peak of COVID-19 cases in the U.S. at the county level, based on the effectiveness of and time to implement containment measures. The tool allows for some user input and scenario modeling.
Dalla Lana School of Public Health, University of Toronto — COVID-19 Growth Model
The goal of this model is to provide insight into the changing nature of case findings and epidemic growth.
COVID Act Now — Hospital Overload Projections for State/County Level
The U.S. Intervention Model is a data platform that projects COVID-19 infections, hospitalizations and deaths across the U.S., as well as how public health interventions contain the spread of COVID-19. It helps decision-makers understand when and how COVID-19 will affect their communities so that they can make better decisions that save lives.