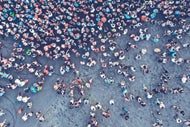
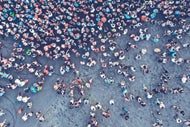
Cleveland Clinic Algorithms Improve Population Health Outcomes
Using technology like artificial intelligence and data to target at-risk and vulnerable populations is still at a nascent stage, but progress is being made. The population health team at Cleveland Clinic has developed a risk-stratification algorithm to help identify those in need of resources, leading to sharp reductions in hospitalization rates among high-risk patients with comorbidities.
The organization uses 120 variables from electronic health records (EHRs), claims data, labs and other results to determine the likelihood that someone would encounter a problem, notes a recent Healthcare Finance report.
Algorithms help the organization deal with an overwhelming amount of data, process the information for meaningful changes in patients’ conditions and automate certain activities, giving well-defined instructions that can be implemented by computers. The algorithms also help clinicians develop effective interventions after analyzing data.
One intervention in the care-at-home division among high-risk patients with comorbidities led to a 35% drop in hospitalizations. The team evaluated patients to determine appropriate interventions and used a service that goes to patients’ homes to help them with connected devices that feed data into the EHR.
The algorithms also help identify gaps in patient assessments. One effort aimed at increasing tighter ambulatory care management of at-risk patients led to a 9.5% increase in what Adam Myers, chief of population health for the Cleveland Clinic, calls “ambulatory touches.” During that same year, in a control population of about 100,000 Medicare patients, inpatient stays dropped 7.5%.
The algorithms also are helping to better manage diabetes patients and others, which is critically important in the effort to advance population health.